Introduction
Deploying AI solutions can revolutionize your business by automating processes, enhancing decision-making, and delivering personalized customer experiences. However, while the potential of AI is immense, many organizations face significant challenges during implementation that can limit its impact. To unlock the full value of AI, it’s crucial to understand and overcome these technical hurdles.
In this blog post, we’ll explore the common challenges businesses encounter when deploying AI solutions and share strategies to help you create an environment where innovation, efficiency, and customer satisfaction thrive.
Key Challenges in Implementing AI Solutions and How to Overcome Them
Implementing AI solutions offers tremendous benefits, such as streamlined processes, data-driven decision-making, and personalized customer experiences. However, these advantages are often accompanied by significant challenges that can complicate the integration process. Common obstacles include data quality issues, talent shortages, and concerns over ethical standards. Successfully navigating these hurdles requires careful planning, organizational commitment, and strategic solutions.
To fully unlock the transformative potential of AI, it’s essential to identify and address these challenges proactively. Here’s a closer look at the key challenges in implementing AI solutions and how to overcome them effectively:
1. Data Quality and Accessibility:
A major challenge in AI deployment is ensuring that the data is high-quality and easily accessible. AI models rely on vast amounts of relevant, accurate, and up-to-date data to function effectively. Yet, many organizations face difficulties with siloed data spread across various systems, inconsistent data standards, and data quality issues like missing values and inaccuracies.
Solutions
- Data Governance: Develop a robust data governance framework with clear standards for data collection, storage, and quality management to ensure data integrity.
- Data Integration Tools: Use data integration tools to consolidate and cleanse data from multiple sources, enabling seamless access and enhancing the performance of AI models
Looking for AI-Fueled Predictive Analytics and Insights?
Tap into the power of predictive analytics with AlphaBOLD. Our AI solutions identify patterns in your business data that reveal new growth opportunities, streamline logistics, and improve customer engagement strategies, giving you a competitive advantage.
Request a Demo2. Model Selection and Training:
Selecting the right AI model is essential for successful integration. The choice of model depends on factors such as the specific problem you’re solving, the quality and quantity of available data, and the desired outcomes. Additionally, training AI models can be resource-intensive, requiring substantial computational power and specialized expertise.
Solutions:
- Proof of Concept (PoC): Run small-scale PoCs to test different AI models and determine which one best fits your needs, reducing risks before full deployment.
- Transfer Learning: Use pre-trained models to cut down on training time and computational demands, making the process more efficient.
- Cross-Functional Teams: Assemble teams of data scientists, domain experts, and engineers to collaboratively select, train, and refine AI models for more effective implementation.
3. Scalability and Infrastructure:
Scalability is a key challenge in AI deployment, especially when dealing with large datasets or complex deep-learning models that demand significant computational power. Your infrastructure must be capable of supporting seamless growth while maintaining high performance.
Solutions:
- Cloud Computing: Leverage cloud platforms that provide scalable computing resources, allowing you to scale up or down as needed without the expense of maintaining extensive on-premises infrastructure.
- Hybrid Architecture: Adopt a hybrid architecture that combines on-premises and cloud resources, ensuring flexibility, optimal performance, and cost efficiency while supporting both current and future AI workloads.
4. Integration with Existing Systems:
Integrating AI solutions with existing legacy systems can be challenging due to differences in architectures, data formats, and communication protocols. Ensuring seamless integration is crucial for leveraging AI capabilities without disrupting current operations.
Solutions:
- APIs and Middleware: Utilize application programming interfaces (APIs) and middleware to bridge communication between AI models and legacy systems, ensuring smooth data exchange and interoperability.
- Microservices: Implement a microservices architecture to facilitate modular integration, allowing new AI functionalities to be added without causing major disruptions to existing workflows.
5. Security and Privacy Concerns:
AI systems often handle sensitive data, making them susceptible to security breaches and privacy violations. Additionally, AI models can inherit biases from the data they are trained on, potentially leading to unfair or discriminatory outcomes.
Solutions:
- Encryption and Access Control: Strengthen data security by implementing robust encryption and access control measures to safeguard sensitive information.
- Bias Mitigation: Regularly audit AI models for biases and use techniques like adversarial training to reduce the risk of biased or discriminatory results.
- Privacy-Preserving Computation: Utilize privacy-preserving methods such as differential privacy and federated learning to ensure data privacy while maintaining model performance.
6. Talent Gap:
The AI talent gap remains a significant barrier to successful integration. Finding professionals with the right mix of technical and domain expertise is challenging.
Solution:
- Upskilling and Training: Invest in upskilling current employees through training programs and certifications.
- Collaborations: Partner with academic institutions and AI research labs to access emerging talent and knowledge.
Get Scalable AI Deployment Across Departments with AlphaBOLD!
Get Scalable AI Deployment Across Departments with AlphaBOLD!
Work with AlphaBOLD to scale AI applications across departments after successful pilot projects. We ensure efficient scaling and optimize resource allocation and performance to maximize ROI while managing implementation costs.
Conclusion
Organizations that successfully integrate AI can achieve substantial business value despite the challenges. By proactively addressing data quality, model selection, scalability, system integration, security, and talent gaps, businesses can overcome technical hurdles and realize the transformative potential of AI. Strategic planning, collaborative efforts, and continuous improvement are key to staying ahead in this dynamic field.
Explore Recent Blog Posts
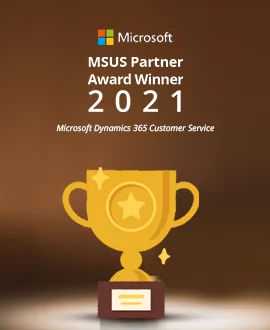