While Artificial Intelligence (AI) fuels progress across industries, the escalating dependence on these systems necessitates robust AI ecosystem measures to shield them from cyberattacks. AI systems introduce unique vulnerabilities that traditional cybersecurity approaches might not cover. In this article, we’ll explore essential AI ecosystem cybersecurity strategies to ensure these systems remain resilient against evolving cyber threats.
Understanding the AI Ecosystem
Explore Our AI Security Solutions
Ready to take your AI security to the next level? Explore our tailor-made AI security solutions designed for your business needs.
Request a ConsultationKey Cybersecurity Measures for AI Implementation
1. Robust Data Protection:
Data is the backbone of AI systems, and securing this data is critical for maintaining trust in AI outputs. Ensuring the integrity and confidentiality of data through encryption, strict access controls, and secure storage are essential first steps in creating a secure AI ecosystem. Encryption helps protect sensitive data both at rest and in transit, while multi-factor authentication (MFA) and role-based access controls (RBAC) limit unauthorized access to critical data points .
In the context of AI ecosystem cybersecurity, protecting data is essential not only for regulatory compliance, such as the General Data Protection Regulation (GDPR) in Europe, which imposes stringent privacy requirements on how personal data is handled, but also to ensure the reliability of AI models. Any compromise in data can severely impact AI performance, leading to skewed results, model drift, or, worse, intentional manipulation by adversaries in the form of data poisoning attacks.
Recent studies have shown that AI models are highly susceptible to attacks targeting their training data. For instance, adversarial manipulations, even subtle changes in input data, can mislead AI algorithms and produce incorrect predictions . Data protection, therefore, is not just about securing information but also about safeguarding the AI models that depend on this data to function optimally.
Moreover, secure storage practices, such as using zero-trust architectures and distributed storage solutions, can mitigate the risks of single-point failures and insider threats . By employing secure data storage, AI systems can continue to function reliably while ensuring that sensitive information is well-protected from unauthorized access or alteration.
2. AI-Specific Security Assessments:
Traditional cybersecurity assessments often fail to account for the unique vulnerabilities that AI systems introduce, such as adversarial attacks, model theft, and data poisoning. Performing AI-specific security assessments is crucial to addressing these risks. These assessments involve AI-focused threat modeling, which helps identify potential attack vectors within the AI lifecycle—from data collection and model training to deployment and real-time inference. Additionally, vulnerability detection techniques, such as penetration testing and adversarial testing, can simulate attacks to evaluate how well the AI system holds up under pressure.
One key aspect of AI-specific assessments is the focus on model interpretability and transparency. Since AI models often operate as “black boxes,” ensuring that they are interpretable helps organizations identify where vulnerabilities may exist, such as unintentional biases or vulnerabilities to adversarial manipulation . Furthermore, incorporating adversarial robustness testing allows organizations to strengthen their models by training them to resist adversarial attacks, ensuring greater resilience .
Such targeted assessments are critical to ensuring comprehensive AI ecosystem cybersecurity. Without them, organizations risk exposing their AI systems to a range of sophisticated attacks that traditional assessments may overlook. Given the growing reliance on AI in decision-making processes, regularly performing these specialized assessments is essential for maintaining trust, reliability, and security in AI systems.
3. Secure AI Development Lifecycle:
Discover AI Opportunities
Unlock the potential of AI for your business. Contact us today to discover how our tailored AI solutions can transform your operations.
Request a Consultation4. Adversarial Training & Testing:
In addition to adversarial training, implementing adversarial testing—which involves intentionally introducing adversarial examples into the system—can further enhance a model’s robustness. This process helps simulate real-world attack scenarios, allowing developers to identify weaknesses in the AI model and improve its defenses. Techniques such as robust optimization and gradient masking can be used to make models more resistant to adversarial manipulations.
Furthermore, ensemble learning methods, which combine multiple models, can reduce the risk of any single model being vulnerable to adversarial attacks. By continuously testing and refining AI models against adversarial inputs, organizations can significantly reduce the likelihood of these attacks causing critical failures in AI systems, ensuring stronger AI ecosystem cybersecurity.
Read more: Top API testing tools for Developers
5. Ethical AI & Governance:
To further fortify AI ecosystem cybersecurity, organizations should implement robust ethical AI guidelines and governance frameworks that ensure responsible use of AI technologies. This includes mandating transparency in AI decision-making, which helps users understand how and why AI systems arrive at certain conclusions, thus reducing the “black box” nature of many AI models. Reducing biases is another critical aspect, as biased algorithms can lead to unfair outcomes, undermining both the security and integrity of AI systems. Techniques like algorithmic fairness and bias detection should be employed to mitigate these risks. Additionally, setting clear accountability measures ensures that there is organizational responsibility for AI decisions, especially in high-stakes environments such as healthcare or finance. According to the European Commission’s Ethics Guidelines for Trustworthy AI, ethical AI practices enhance trust and security, safeguarding both users and AI ecosystem cybersecurity from misuse or harmful outcomes. Moreover, the OECD’s AI Principles highlight the importance of fairness, transparency, and accountability as key pillars in building secure and trustworthy AI ecosystems.
6. Collaboration & Knowledge Sharing:
Conclusion
Secure your AI ecosystem today
Schedule a consultation to protect your systems from emerging cyber threats and stay ahead with cutting-edge AI security solutions.
Request a ConsultationExplore Recent Blog Posts
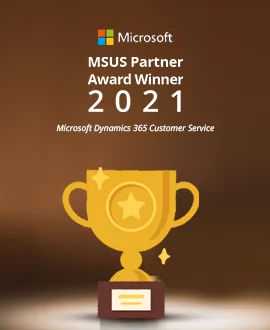