Table of Contents
Introduction
This blog will explore the practical benefits of AI-powered predictive maintenance, including cost savings, increased equipment reliability, and improved operational efficiency. We’ll also look at real-world examples of its application in manufacturing and address the challenges organizations might face when implementing such systems. By the end, you’ll clearly understand how this technology can enhance your operations and what it takes to adopt it effectively.
Manufacturing depends on the seamless operation of machinery to meet production demands and ensure quality. However, unexpected equipment failures can bring production lines to a standstill, resulting in significant financial losses—often reaching as high as $260,000 per hour of downtime. Traditional maintenance strategies, such as reactive and preventive approaches, are often inefficient. Reactive maintenance addresses problems only after they occur, while preventive maintenance adheres to fixed schedules that may not reflect actual equipment conditions.
AI- based predictive maintenance offers a forward-looking alternative. This approach identifies patterns that indicate potential equipment issues by leveraging sensor data, historical maintenance logs, and operational records. Advanced machine learning models and techniques, such as anomaly detection and time-series analysis, enable manufacturers to predict failures precisely, reducing unplanned downtime and optimizing maintenance schedules.
Benefits of AI-Powered Predictive Maintenance in Manufacturing
Manufacturers face significant challenges with traditional maintenance methods. Reactive maintenance addresses equipment issues after a failure and is costly and disruptive. According to a report by Deloitte, unplanned downtime costs industrial manufacturers an estimated $50 billion annually, with maintenance expenses making up a large portion of these losses. Similarly, scheduled preventive maintenance, while preemptive, often results in the premature replacement of parts and unnecessary servicing, leading to wasted resources and inflated costs.
In contrast, advanced maintenance strategies leverage machine learning (ML), deep learning, and advanced analytics. This approach relies on real-time equipment monitoring through sensors and operational data to predict faults before they escalate into critical issues. Below, we’ll explore the key benefits of this innovative approach.
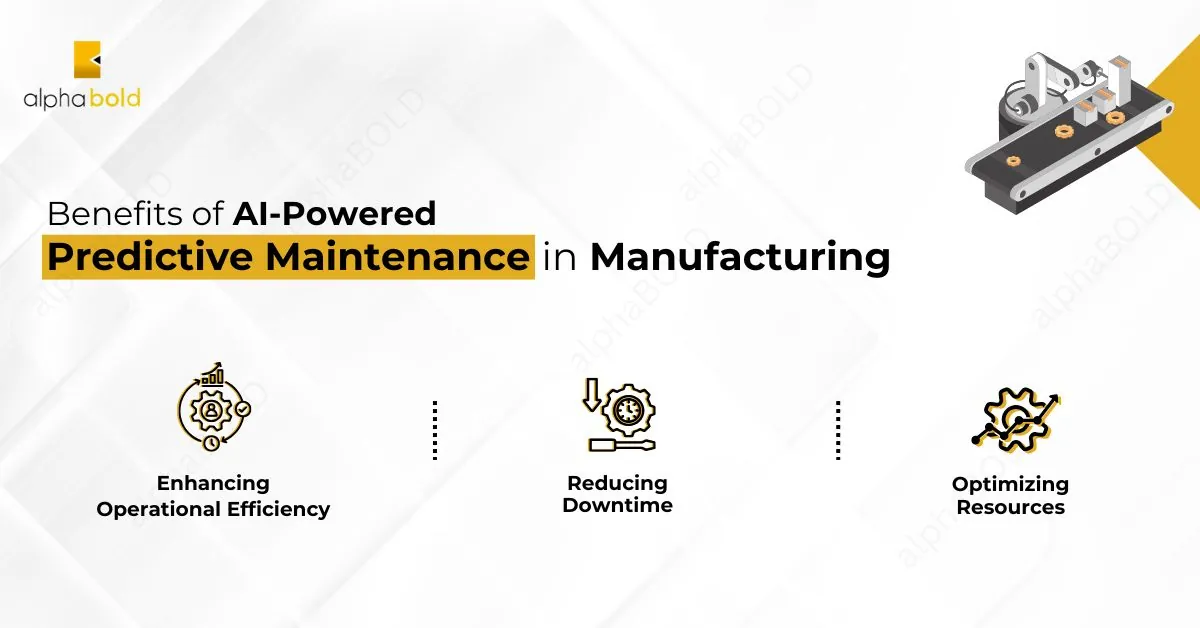
You may also like: 4 Key Steps for Business Leaders to Implement AI
1. Enhancing Operational Efficiency:
Operational efficiency is a top priority for manufacturers, and AI-powered predictive maintenance is crucial in achieving this. By detecting equipment issues early, manufacturers can prevent small problems from escalating into costly failures, ensuring production schedules remain on track. AI models continuously learn and adapt to new data, enabling more accurate forecasts and timely interventions.
For example, a study by McKinsey found that predictive maintenance can decrease machine uptime by 20% to 40%, translating into significant productivity gains. Maintenance teams can shift their focus from routine inspections of functioning equipment to addressing critical and high-priority tasks and optimizing labor and resources. This targeted maintenance approach improves operational efficiency and allows manufacturers to allocate resources where they are most needed.
2. Reducing Downtime:
Unplanned downtime is a costly problem that can be avoided by using AI in the manufacturing sector. Studies show that 82% of companies have experienced unplanned downtime over the past three years, with downtime lasting an average of four hours per incident. AI predictive maintenance provides early failure alerts, enabling manufacturers to address issues before they disrupt operations.
For instance, an unexpected increase in machine vibration might indicate misalignment or bearing issues. AI systems detect these anomalies in real-time and notify maintenance teams, who can take corrective action during planned downtime. This approach significantly reduces unscheduled downtime, safeguards production schedules, and minimizes revenue losses.
A real-world example comes from Siemens, which implemented predictive maintenance in its production lines. The company reported a 30% reduction in maintenance costs and a 50% decrease in downtime, demonstrating the potential savings for manufacturers who adopt AI-driven maintenance.
3. Optimizing Resources:
Resource optimization is another significant advantage of predictive maintenance. Traditional maintenance strategies often lead to over-maintenance, wasting spare parts, labor, and time. Predictive maintenance ensures that service is performed only when necessary, reducing waste and extending the life of equipment.
AI can also predict the demand for spare components by analyzing historical usage patterns and operational data. For example, an AI system might forecast the replacement schedule for machine bearings, helping manufacturers maintain optimal inventory levels. This prevents overstocking, reduces carrying costs, and improves supply chain efficiency.
Additionally, AI-powered predictive maintenance contributes to energy efficiency. If a motor begins consuming more power than usual, AI systems can identify the issue as a sign of wear and tear. Addressing such inefficiencies promptly reduces energy consumption and operational expenses. According to the U.S. Department of Energy, predictive maintenance can improve energy efficiency by up to 20%.
Optimize Your Maintenance Strategy Today
Discover how advanced maintenance solutions can save costs, minimize downtime, and enhance equipment reliability. Let our experts guide you through implementing tailored solutions to meet your unique needs.
Request a ConsultationReal-World Applications of AI-Powered Predictive Maintenance
AI-based predictive maintenance is critical for enhancing efficiency, reliability, and cost savings across various industries. Here are some notable applications and examples that highlight their transformative potential:
1. Automotive Industry: Ensuring Assembly Line Reliability:
The automotive industry relies heavily on automated assembly lines, where downtime can disrupt production schedules and incur significant costs. Predictive maintenance fueled by AI plays a vital role in monitoring robotic arms, conveyors, and other critical machinery to predict potential failures.
For example, Ford Motor Company implemented AI-driven predictive maintenance across its manufacturing plants. By analyzing sensor data from robotic systems, the company identified wear patterns and potential failure in real-time, reducing unexpected downtime and improving overall production efficiency (Source: Ford Annual Manufacturing Report).
2. Aerospace: Enhancing Safety and Operational Efficiency:
In aerospace, safety is paramount, and predictive maintenance ensures aircraft components function reliably. AI systems analyze data from jet engines, hydraulic systems, and landing gear to detect anomalies early. By predicting failures, maintenance can be scheduled during routine ground times, avoiding disruptions to flight schedules.
Delta Airlines, for instance, uses predictive maintenance tools powered by AI to monitor its fleet. The airline reported a 98% reduction in in-flight component failures after integrating these systems, which analyze engine performance data to predict issues before they become critical.
3. Energy and Utilities: Optimizing Power Generation and Supply:
Energy producers, including power plants and renewable energy facilities, depend on predictive maintenance to ensure equipment like turbines, generators, and transformers operate smoothly. AI systems continuously monitor temperature, vibration, and pressure to identify potential problems.
A prominent example is GE Renewable Energy, which uses AI-driven predictive maintenance for wind turbines. The system identifies unusual vibration patterns that might indicate bearing failures, allowing preemptive action. GE reported a 20% reduction in maintenance costs and a 10% improvement in turbine uptime, leading to more efficient energy generation (Source: GE Energy Insights).
4. Food and Beverage Industry: Ensuring Quality and Reliability:
Equipment reliability is critical for maintaining production quality and preventing spoilage in the food and beverage sector. Predictive maintenance monitors parameters like temperature, pressure, and mechanical wear to ensure that processing and packaging machines perform optimally.
For example, Nestlé deployed predictive maintenance systems in its production facilities to monitor key metrics in real-time. The company reduced unplanned downtime and enhanced overall equipment effectiveness. These systems also help maintain strict compliance with food safety regulations by preventing equipment malfunctions that could impact product quality.
These real-world examples of AI-powered predictive maintenance demonstrate its ability to improve efficiency, reduce costs, and enhance safety. As adoption grows, companies embracing predictive maintenance can gain a significant edge in their respective industries.
Imagine reducing maintenance costs and preventing unexpected breakdowns with a reliable, data-driven system!
AlphaBOLD’s AI consultants specialize in predictive maintenance solutions that deliver measurable results. Get started—book your consultation now.
Request a ConsultationImplementation Challenges of AI-Powered Predictive Maintenance for Manufacturers
While AI-driven predictive maintenance offers transformative benefits, its implementation comes with challenges that manufacturers must address to savor its potential. Here are the key obstacles and strategies for overcoming them:
1. Legacy System Integration:
Many manufacturing facilities still rely on older equipment that lacks the necessary sensors or connectivity for predictive maintenance. Retrofitting legacy machines with IoT devices can be complex and costly, often requiring significant modifications to integrate them into modern predictive maintenance systems.
For example, a manufacturing plant with decades-old machinery may need custom-built sensors and connectivity solutions to gather real-time data. However, manufacturers can prioritize retrofitting critical equipment first, gradually scaling their predictive maintenance capabilities.
2. Data Quality and Accessibility:
AI models rely on vast amounts of high-quality data for accurate predictions. However, fragmented systems, poor data storage practices, and inconsistent data formats often hinder data usability. Without reliable, clean, and well-organized data, AI algorithms may produce inaccurate or irrelevant insights.
To address this challenge, manufacturers should invest in robust data collection and preprocessing systems, such as centralized data lakes and advanced analytics platforms. For example, companies like Siemens have successfully implemented AI-based predictive maintenance by combining IoT-enabled devices with high-performance data management systems.
3. Skill Gaps in the Workforce:
Implementing and managing AI-powered predictive maintenance systems requires expertise in AI, machine learning, and data analytics. Many manufacturers face a shortage of skilled personnel capable of interpreting data, managing AI models, and making informed decisions.
Upskilling existing teams through targeted training programs and hiring specialized talent can help bridge this gap. Additionally, partnering with AI consultants can provide the expertise needed to implement these systems effectively, ensuring long-term success.
4. Cybersecurity Risks:
As manufacturing facilities become increasingly connected, they also become more vulnerable to cyberattacks. Breaches can compromise sensitive operational data, disrupt production processes, and damage system integrity.
To mitigate these risks, manufacturers must implement robust cybersecurity measures, such as end-to-end encryption, secure data storage, and continuous network monitoring. For instance, adopting AI-based threat detection systems can proactively identify and neutralize security risks in real time.
How to Navigate These Challenges?
Overcoming these challenges requires careful planning, the right expertise, and customized solutions. This is where AlphaBOLD can help. As a leader in AI and digital transformation, AlphaBOLD specializes in designing and implementing customized AI-powered predictive maintenance systems for manufacturers.
Through their comprehensive AI solutions, AlphaBOLD helps manufacturers:
- Integrate predictive maintenance solutions with legacy systems.
- Build data pipelines to ensure high-quality, actionable insights.
- Upskill teams through training programs and provide ongoing technical support.
- Enhance cybersecurity to protect critical data and maintain system integrity.
AlphaBOLD’s AI consultants ensure that manufacturers not only implement predictive maintenance effectively but also maximize its benefits for improved efficiency, reduced downtime, and sustainable operations.
Conclusion
Predictive maintenance powered by data-driven insights is quickly becoming essential for manufacturers looking to reduce downtime, improve efficiency, and cut costs. By identifying potential equipment failures before they happen, businesses can maintain smoother operations, meet production targets, and enhance their bottom line.
Implementing this approach requires thoughtful planning and effort. From integrating modern solutions with older equipment to ensuring data accuracy, bridging skill gaps, and protecting systems from cyber threats, the challenges are real but manageable.
Ready to minimize downtime and maximize efficiency?
Let’s discuss how AI-powered predictive maintenance can transform your manufacturing operations. Request a consultation with our experts at AlphaBOLD and discover a solution customized to your needs.
Request a ConsultationExplore Recent Blog Posts
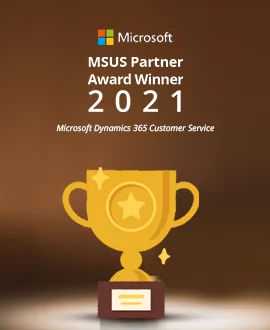