Table of Contents
Introduction
In 2024 alone, the global artificial intelligence market reached an estimated valuation of $200 billion, with projections to surpass $826.70 billion by 2030. While businesses continue adopting AI-driven solutions, one transformative innovation remains underutilized Agentic AI. Unlike traditional generative AI, which creates content or automates tasks, Agentic AI plans, executes, and adapts autonomously, redefining decision-making and operational efficiency.
Nowhere is this evolution more impactful than in Retrieval-Augmented Generation (RAG) applications. RAG systems already bridge real-time information retrieval with generative AI, but they often struggle with contextual understanding, adaptability, and decision-making. Agentic AI enhances RAG applications by enabling deeper contextual intelligence, autonomous learning, and actionable decision support—giving businesses a true competitive edge.
In this blog, we’ll explore how Agentic AI powers next-gen RAG applications, transforming them from passive retrieval models into intelligent, adaptive, and self-improving systems that drive efficiency, accuracy, and scale.
Understanding RAG Applications: Bridging Retrieval and Generation
Retrieval-Augmented Generation (RAG) is an AI framework that combines information retrieval with generative AI to enhance the accuracy and relevance of responses. Unlike traditional generative models that rely solely on pre-trained knowledge, RAG applications fetch real-time data from external sources—such as databases, APIs, or the web—before generating responses.
This approach is widely used in chatbots, enterprise search engines, customer service automation, and knowledge management systems, allowing businesses to deliver more context-aware, accurate, and up-to-date insights in real-time.
Agentic AI: Your Self-sufficient AI system
Agentic AI is a self-directed AI system designed to function autonomously. Unlike traditional AI, it does not require pre-programmed instructions or human intervention. Agentic AI operates with cognitive autonomy; it strategizes and executes tasks. Consider it as an intelligent agent that can assess complex scenarios, make informed decisions, and dynamically adapt to shifting environments.
Envision managing a portfolio during a global economic crisis. Traditional AI might identify declining asset values but fail to assess the broader implications. An Agentic AI system, powered by Large Language Models (LLMs) and contextual learning, evaluates every factor in play:
- Market Volatility: Uses historical patterns to predict potential price recovery or further decline.
- Global Events: Assesses news sentiment (via natural language processing) to understand the drivers behind the market shift.
- Risk Scenarios: Simulates potential outcomes for various investment strategies, advising which assets to hold, sell, or reinvest in.
As a result, you’ll get an intelligent, adaptive system that minimizes losses and seizes opportunities, essentially acting as a financial advisor that never sleeps.
Explore further: Selecting a Tech Partner for AI Project
Set Your AI Project Up for Success!
Make sure your organization is fully prepared for your upcoming AI project. Download our comprehensive checklist.
Download NowAgentic AI Powers Smarter RAG Applications
Before exploring how Agentic AI transforms RAG (Retrieval-Augmented Generation) applications, let’s establish some context. RAG is a methodology that combines information retrieval systems with generative AI models, creating a hybrid that excels at handling large datasets. Instead of relying solely on static training data, RAG retrieves real-time, contextually relevant information from external knowledge sources (e.g., databases, APIs, or the internet) to enhance generative outputs.
In practical terms, RAG applications aim to bridge the gap between knowledge retrieval and natural language generation. Examples include:
- Chatbots that generate answers based on dynamic data.
- Enterprise tools that provide contextual recommendations by pulling from internal documents.
- Customer service applications that retrieve real-time policy updates to answer complex queries.
While RAG systems have already proven their value, they often face challenges like shallow contextual understanding, static data dependencies, and limited adaptability. All these gaps are directly catered with Agentic AI.
How Agentic AI Enhances RAG Applications
1. Enhanced Contextual Understanding:
Traditional RAG systems often retrieve data based on simple keyword matches or predefined rules, which can lead to irrelevant or incomplete outputs. Agentic AI, powered by context-aware algorithms, significantly improves the relevance of retrieved data.
How It Works: Agentic AI uses transformer-based architectures (like those found in LLMs) to analyze a query’s nuances. It evaluates the query context, intent, and even subtleties like tone or ambiguity to retrieve the most appropriate information.
Example: Imagine querying a RAG app for “marketing strategies.” Traditional RAG might return to a list of unrelated articles. Agentic AI identifies whether you mean digital marketing for startups or corporate B2B strategies and curates precise, actionable insights accordingly.
2. Dynamic Learning and Refinement:
A common limitation of RAG systems is their reliance on static datasets and pre-defined rules. Agentic AI overcomes this by employing Continuous Learning Models (CLMs) that refine their understanding of queries over time. Every interaction improves the system’s performance.
How It Works: With reinforcement learning, Agentic AI adapts based on user feedback, historical queries, and changes in data patterns. Over time, it becomes better at predicting user needs and retrieving more targeted information.
Example: A business intelligence platform powered by Agentic AI learns from each query about financial data, gradually improving its ability to predict what metrics decision-makers will prioritize during future interactions.
3. Autonomous Decision-Making:
Traditional RAG systems stop at retrieval. They present raw data, leaving the user to extract insights and act. Agentic AI takes this further by combining retrieval with actionable decision-making.
How It Works: Agentic AI evaluates the retrieved data and generates recommendations or decisions based on predefined business goals or user contexts. It uses decision-support frameworks to suggest optimal actions autonomously.
Example: In sales forecasting, an Agentic AI-powered RAG application retrieves last quarter’s sales data and analyzes it in real-time. It forecasts upcoming trends and recommends specific inventory adjustments or pricing changes.
4. Personalization for Superior User Experience:
One of the standout advantages of Agentic AI in RAG applications is its ability to deliver personalized outputs. By analyzing user behavior, preferences, and past interactions, Agentic AI tailors responses to fit individual needs.
How It Works: Agentic AI fine-tunes its outputs by leveraging user data and behavioral analytics to align with unique preferences.
Example: A customer support chatbot powered by Agentic AI not only answers FAQs but also remembers past issues reported by the user. The chatbot provides solutions tailored to their concerns, improving customer satisfaction and loyalty.
Achieve Seamless AI Integration Across Departments with AlphaBOLD!
Scale your AI success with AlphaBOLD—expand AI solutions across your organization after a successful pilot.
Request a ConsultationConclusion
In conclusion, Agentic AI empowers Retrieval-Augmented Generation (RAG) systems with cognitive autonomy, transforming them from passive information retrieval tools to proactive, decision-support systems.
By leveraging advanced reasoning, contextual learning, and multi-agent collaboration, Agentic AI enhances RAG applications, enabling deeper contextual understanding, dynamic adaptation, and autonomous decision-making. This empowers organizations to unlock RAG’s full potential—boosting operational efficiency, agility, and competitive advantage.
To successfully deploy these capabilities within your organization, partnering with experienced AI specialists is crucial. AlphaBOLD’s expert AI engineers provide the strategic guidance and technical expertise needed to seamlessly integrate Agentic AI, ensuring scalable and impactful implementation.
Explore Recent Blog Posts
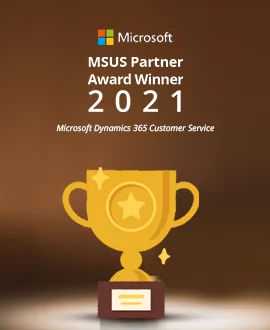